Overview of Machine Learning Frameworks for Analyzing Animal Behavior
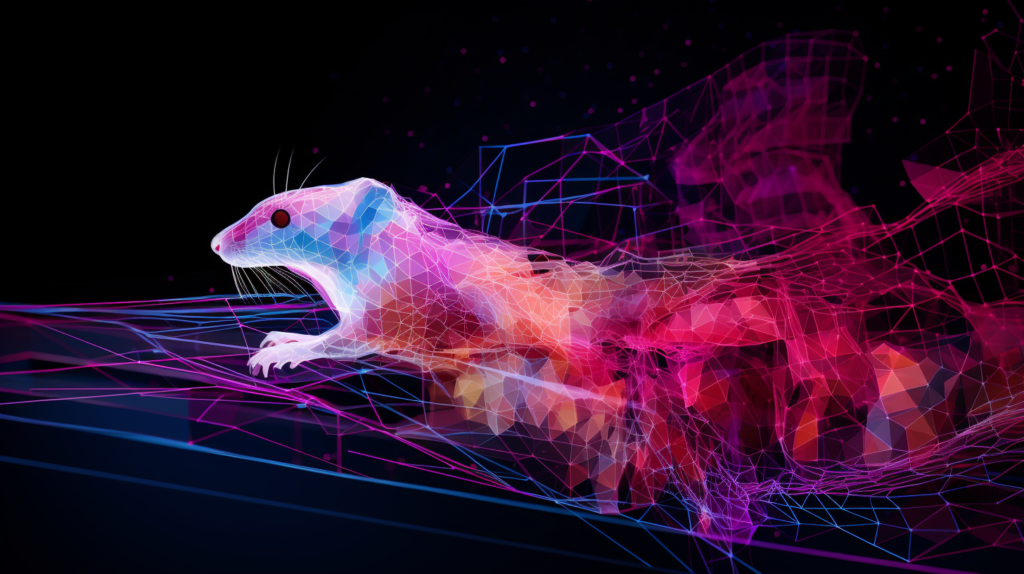
Introduction to Machine Learning Frameworks for Studying Animal Behavior
Open-source ML frameworks have emerged as powerful tools in animal behavioral studies, offering researchers a blend of accessibility, flexibility, and advanced analytical capabilities. This page provides an overview of commercial and open-source machine learning frameworks commonly used in biomedical research.
Beyond cost-effectiveness, open-source tools promote innovation through collaboration and transparency in research.
Aivero aims to make video capturing easily connected with state-of-the-art machine learning models to improve research efficiency.
Machine Learning Techniques and Algorithms
Machine learning (ML) has become a pivotal tool in the study of animal behavior, leveraging video data to offer deeper insights into the complex world of animal dynamics. Machine learning models, particularly those using deep learning techniques like Convolutional Neural Networks (CNNs), are trained on vast datasets to recognize and categorize different environments and scenarios depicted in videos. This capability is crucial in fields where the system must accurately interpret conditions and categorization.
By applying advanced ML algorithms to video footage, researchers can automate the process of tracking and analyzing animal movements and interactions, which was traditionally a labor-intensive task. ML models, particularly those using deep learning, are trained to recognize and differentiate between various animal species, individual animals, and even specific behaviors.
Another significant advancement brought by ML in studying animal behavior is the ability to analyze large-scale video datasets over extended periods. This longitudinal analysis allows for the observation of behavioral changes over time, contributing to long-term studies on animal development, adaptation, and the impact of environmental changes. Machine learning algorithms are also adept at working with varying qualities of video data, from high-resolution lab recordings to lower-quality field footage, making them versatile tools in diverse research settings. Because of these variations it is important to choose the correct camera for your application.
Additionally, the integration of unsupervised learning techniques helps in discovering new behavioral patterns without pre-defined classifications, leading to novel insights. For example, unsupervised algorithms can cluster different behaviors based on visual similarities, unveiling previously unrecognized patterns or categorizing complex social interactions. This automated, data-driven approach not only enhances the accuracy and efficiency of behavioral analysis but also opens up new avenues for exploration in animal behavior research.
Overview of Machine Learning Frameworks
In the following table, we are continuously adding new ML frameworks for researchers doing animal behavior research:
(Missing a framework? Let us know using this form)